The Ultimate Guide to Image Classification Methods
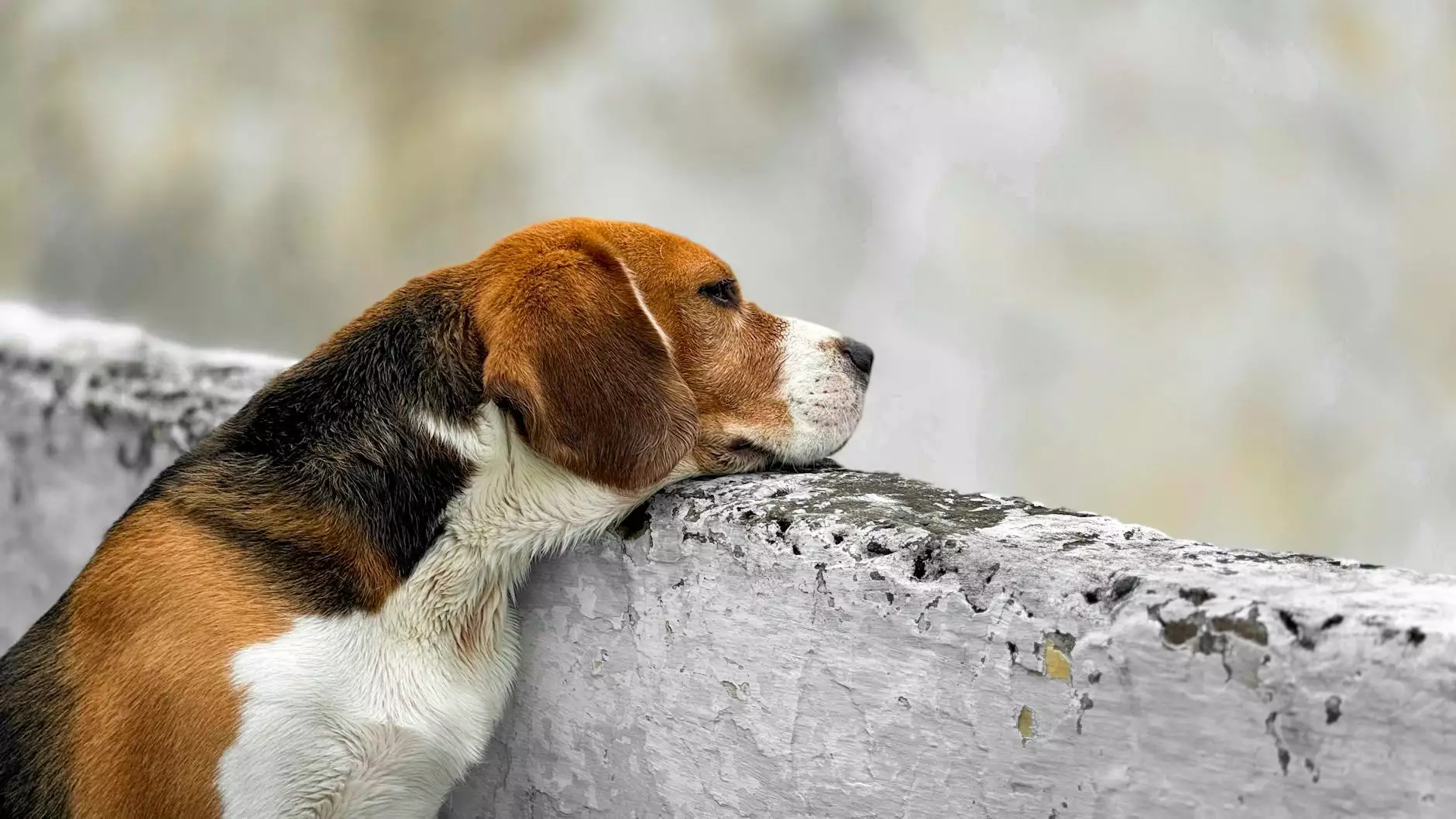
In today's digital age, the significance of image classification methods cannot be overstated. As businesses increasingly rely on visual data, understanding how to effectively classify and interpret this information becomes essential. This article delves into the various image classification methods, their applications, and the role of data annotation tools and platforms, specifically highlighting how Keylabs.ai can enhance your image classification projects.
Understanding Image Classification
Image classification is the process of assigning a label to an image based on its content. It involves analyzing visual data through a combination of machine learning, computer vision, and data annotation techniques. The aim is to identify and categorize objects within an image accurately.
The Importance of Image Classification
Effective image classification is crucial for numerous industries, including:
- Healthcare: Diagnosing diseases from medical images such as X-rays, MRIs, and CT scans.
- Retail: Analyzing customer preferences and enhancing shopping experiences through visual search.
- Autonomous Vehicles: Identifying traffic signs, pedestrians, and road conditions to improve safety.
- Security: Detecting anomalies in surveillance footage for enhanced security measures.
Fundamental Image Classification Methods
A variety of methods are employed in image classification, ranging from traditional algorithms to modern deep learning techniques. Here we explore some of the most prominent image classification methods.
1. Traditional Machine Learning Approaches
Early image classification techniques primarily relied on traditional machine learning algorithms. Key methods include:
- K-Nearest Neighbors (KNN): This algorithm classifies images based on the majority voting system of their nearest neighbors in the feature space.
- Support Vector Machines (SVM): SVMs aim to find the hyperplane that best separates different classes in the feature space.
- Decision Trees: This method uses a tree-like structure to model decisions and their possible consequences.
- Random Forests: An ensemble method that creates a 'forest' of decision trees to improve classification results through majority voting.
2. Deep Learning Techniques
With advancements in technology, deep learning has revolutionized image classification methods. Some popular techniques involve:
- Convolutional Neural Networks (CNNs): These networks are specifically designed for processing structured grid data like images, recognizing spatial hierarchies effectively.
- Transfer Learning: This method involves taking a pre-trained model and fine-tuning it on a specific dataset, allowing for faster training with less data.
- Generative Adversarial Networks (GANs): GANs consist of two networks, a generator and a discriminator, which cooperate to improve the quality of generated images for training.
Challenges in Image Classification
Despite the advancements, several challenges remain in effective image classification:
- Data Quality: Poor data quality leads to inaccurate classification outcomes. High-quality labeled data is crucial.
- Labeling Variability: Inconsistencies in data annotation can significantly impact classification accuracy.
- Scalability: Handling large datasets efficiently while maintaining performance poses a challenge for many businesses.
- Overfitting: Creating a model that performs well on training data but poorly on unseen data is a common issue.
The Role of Data Annotation in Image Classification
Data annotation is a critical step in the image classification process. It involves labeling images with the correct tags or categories that the model will learn to recognize. Efficient data annotation is fundamental for training robust models.
Data Annotation Tools and Platforms
To improve data annotation processes, various tools and platforms have emerged. Below are some key features to look for:
- Ease of Use: An intuitive interface attracts more users, enhancing productivity.
- Collaboration Features: Tools that allow for multi-user access streamline workflow and reduce bottlenecks.
- Integration Capabilities: Compatibility with existing workflows and machine learning frameworks is vital.
- Quality Control Options: The ability to monitor and verify annotations helps maintain accuracy.
How Keylabs.ai Enhances Data Annotation
Keylabs.ai specializes in providing advanced data annotation tools and platforms that aid businesses in streamlining their image classification processes. Here’s how:
1. High-Quality Data Annotation Services
Keylabs.ai offers professional data annotation services with meticulous attention to detail, ensuring high-quality outputs that improve the performance of your image classification models.
2. Versatile Annotation Tools
From bounding boxes to segmentation masks, Keylabs.ai provides a variety of annotation tools that cater to different project requirements, enabling precise labeling for diverse use cases.
3. AI-Powered Solutions
With integrations of AI technology, Keylabs.ai accelerates the annotation process while maintaining a high degree of accuracy, allowing your team to focus on analyzing results rather than managing tedious tasks.
4. Collaborative Platform
Keylabs.ai fosters collaboration among teams, enabling seamless communication and project management. This efficiency ultimately leads to a quicker turnaround time for your projects.
Future of Image Classification Methods
The landscape of image classification is continually evolving. As technologies advance, we can expect to see:
- Improved Algorithms: Ongoing research into more sophisticated models will enhance classification accuracy and efficiency.
- More Accessible Tools: The democratization of AI will make advanced tools available to smaller businesses.
- Ethical Considerations: Rising concern over privacy and bias in AI will drive the development of more ethical image classification practices.
Conclusion
Understanding and implementing effective image classification methods can propel businesses forward, unlocking new opportunities and enhancing operational efficiencies. With powerful data annotation tools and platforms like Keylabs.ai, organizations can harness the full potential of visual data. Investing in quality image classification strategies is not just an option; it’s a necessity for businesses aiming for success in an increasingly data-driven world.